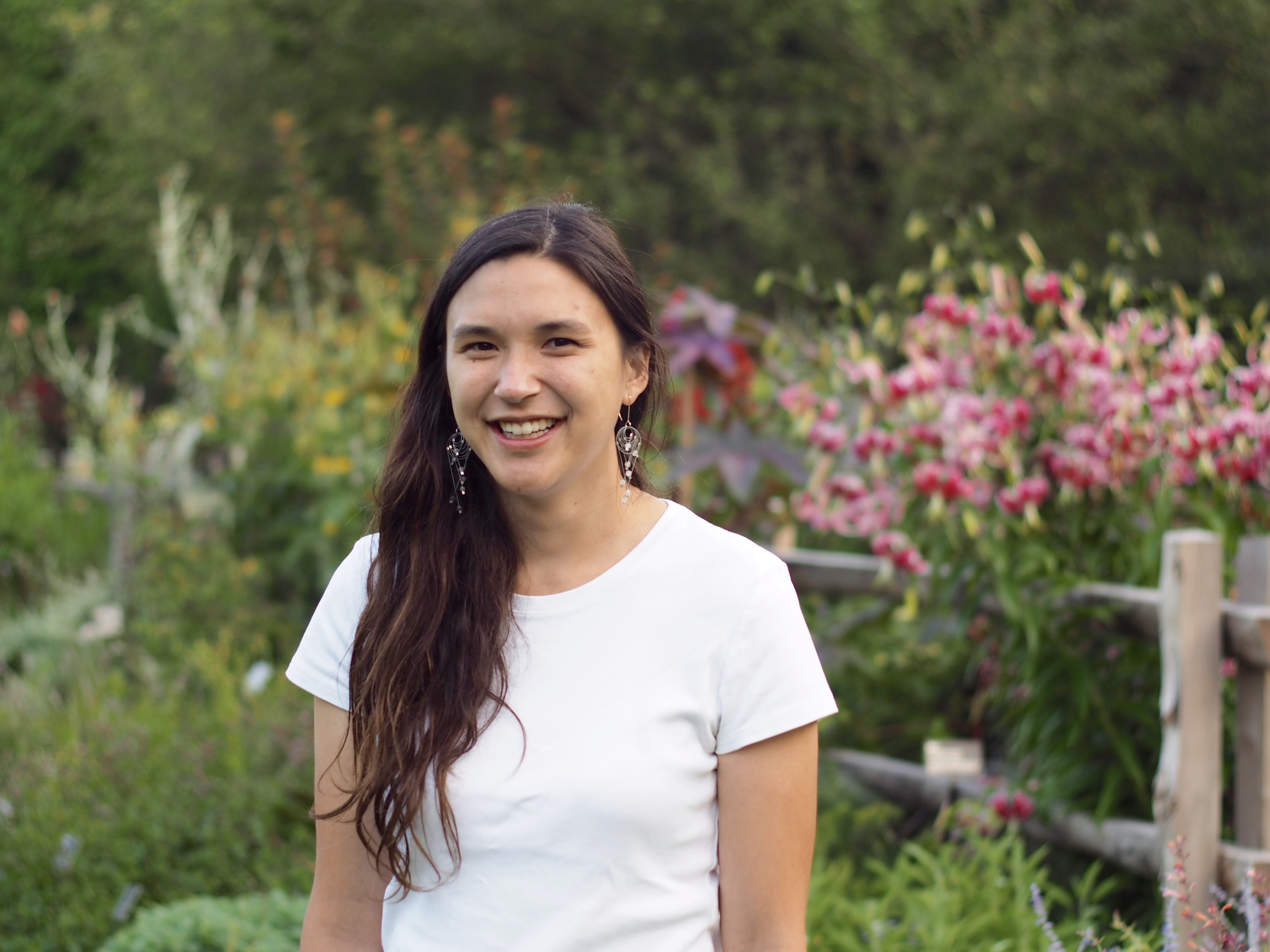
HELLO!
EMAIL: courtney(dot)paquette(at)mcgill(dot)ca
OFFICE: BURN 913
I am an assistant professor at McGill University in the Mathematics and Statistics department. I am a CIFAR AI Chair (MILA) and I am an active member of the Montreal Machine Learning Optimization Group (MTL MLOpt) at MILA. Moreover I am one of the lead organizers of the OPT-ML Workshop at NeurIPS since 2020 and I am one of the lead organizers (and original creator) of the High-dimensional Learning Dynamics (HiLD) Workshop at ICML. I also work 20% as a research scientist at Google DeepMind, Montreal. You can view my CV here if you are interested in more details.
I received my Ph.D. from the Mathematics department at the University of Washington (2017) under Prof. Dmitriy Drusvyatskiy then I held a postdoctoral position in the Industrial and Systems Engineering at Lehigh University where I worked with Prof. Katya Scheinberg. I held an NSF postdoctoral fellowship (2018-2019) under Prof. Stephen Vavasis in the Combinatorics and Optimization Department at the University of Waterloo.
My research broadly focuses on designing and analyzing algorithms for large-scale optimization problems, motivated by applications in data science. The techniques I use draw from a variety of fields including probability, complexity theory, and convex and nonsmooth analysis. I also study scaling limits of stochastic algorithms.
For a magazine article about myself and my research, see REACH Magazine Rising Star in AI, 2022. I also recently received the Sloan Fellowship in Computer Science (2024).
University of Washington, Lehigh University, University of Waterloo, McGill University, and MIlA have strong optimization groups which spans across many departments: Math, Stats, CSE, EE, and ISE. If you are interested in optimization talks at these places, check out the following seminars:
- High-dimensional Learning Dynamics (HiLD) workshop at ICML
- Optimization for Machine Learning (OPT+ML) workshop at NeurIPS
- Montreal Machine Learning and Optimization (MTL MLOPT) at MILA
- Applied Mathematics at McGill University
- Trends in Optimization Seminar (TOPS/CORE) at University of Washington
- Institute for Foundations of Data Science at University of Washington/University of Wisconsin
- Machine Learning at Paul G. Allen School of Computer Science and Engineering, University of Washington
- COR@L at Lehigh University
- Combinatorics and Optimization at University of Waterloo
If you are looking for another mathematician (probabilist) named Paquette, see Elliot Paquette.
RESEARCH
My research interests lie at the frontier of large-scale continuous optimization. Nonconvexity, nonsmooth analysis, complexity bounds, and interactions with random matrix theory and high-dimensional statistics appear throughout work. Modern applications of machine learning demand these advanced tools and motivate me to develop theoretical guarantees with an eye towards immediate practical value. My current research program is concerned with developing a coherent mathematical framework for analyzing average-case (typical) complexity and exact dynamics of learning algorithms in the high-dimensional setting.
You can view my CV here if you are interested in more details.
You can view my thesis titled: Structure and complexity in non-convex and nonsmooth optimization.
RESEARCH PAPERS
Papers are arranged in reverse chronological order, according to the date they are submitted to the arXiv
* indicates student author
- Elliot Paquette, Courtney Paquette, Lechao Xiao, Jeffrey Pennington. 4+3 Phases of Compute-Optimal Neural Scaling Laws. (submitted), 2024, https://arxiv.org/pdf/2405.15074
- Elizabeth Collins-Woodfin, Inbar Seroussi, Begoña García Malaxechebarría*, Andrew Mackenzie*, Elliot Paquette, Courtney Paquette. The High Line: Exact Risk and Learning Rate Curves of Stochastic Adaptive Learning Rate Algorithms. (submitted), 2024, https://arxiv.org/pdf/2405.19585
- Tomás González*, Cristóbal Guzman, Courtney Paquette. Mirror Descent Algorithms with Nearly Dimension-Independent Rates for Differentially-Private Stochastic Saddle-Point Problems. (accepted) 37th Annual Conference on Learning Theory (COLT), 2024, https://arxiv.org/pdf/2403.02912
- Pierre Marion*, Anna Korba, Peter Barlett, Mathieu Blondel, Valentin De Bortoli, Arnaud Doucet, Felipe Llinares-López*, Courtney Paquette, Quentin Berthet. Implicit Diffusion: Efficient Optimization through Stochastic Sampling. (submitted), 2024, https://arxiv.org/pdf/2402.05468
- Elizabeth Collins-Woodfin, Courtney Paquette, Elliot Paquette, Inbar Seroussi. Hitting the High-Dimensional Notes: An ODE for SGD learning dynamics on GLMs and multi-index models. (submitted), 2023, https://arxiv.org/pdf/2308.08977
- Courtney Paquette, Elliot Paquette, Ben Adlam, Jeffrey Pennington. Implicit Regularization or Implicit Conditioning? Exact Risk Trajectories of SGD in High Dimensions. Advances in Neural Information Processing Systems 35 (NeurIPS), 2022, paper link
- Kiwon Lee*, Andrew N. Cheng*, Elliot Paquette, Courtney Paquette. Trajectory of Mini-Batch Momentum: Batch Size Saturation and Convergence in High-Dimensions. Advances in Neural Information Processing Systems 35 (NeurIPS), 2022, paper link
- Courtney Paquette, Elliot Paquette, Ben Adlam, Jeffrey Pennington. Homogenization of SGD in high-dimensions: Exact dynamics and generalization properties. (submitted), 2022, https://arxiv.org/pdf/2205.07069
- Leonardo Cunha*, Gauthier Gidel, Fabian Pedregosa, Damien Scieur, Courtney Paquette. Only Tails Matter: Average-case Universality and Robustness in the Convex Regime. Proceedings of the 39th International Conference on Machine Learning (ICML), 2022, https://proceedings.mlr.press/v162/cunha22a
- Courtney Paquette, Elliot Paquette. Dynamics of Stochastic Momentum Methods on Large-scale, Quadratic Models. Advances in Neural Information Processing Systems 34 (NeurIPS), 2021, paper link
- Courtney Paquette, Kiwon Lee*, Fabian Pedregosa, Elliot Paquette. SGD in the Large: Average-case Analysis, Asymptotics, and Stepsize Criticality. 34th Annual Conference on Learning Theory (COLT), 2021, https://proceedings.mlr.press/v134/paquette21a
- Courtney Paquette, Bart van Merrienboer, Elliot Paquette, Fabian Pedregosa. Halting time is predictable for large models: A Universality Property and Average-case Analysis. Found. Comput. Math. 23 (2023), no.2, 597–673, https://doi.org/10.1007/s10208-022-09554-y
- Sina Baghal, Courtney Paquette, and Stephen A. Vavasis. A termination criterion for stochastic gradient for binary classification. (submitted), 2020, https://arxiv.org/pdf/2003.10312
- Courtney Paquette, Stephen A. Vavasis. Potential-based analyses of first-order methods for constrained and composite optimization. (submitted), 2019, https://arxiv.org/pdf/1903.08497
- Courtney Paquette, Katya Scheinberg. A Stochastic Line Search Method with Expected Complexity Analysis. SIAM J. Optim. 30 (2020), no. 1, 349-376, https://doi.org/10.1137/18M1216250
- Damek Davis, Dmitriy Drusvyatskiy, Kellie J. MacPhee, Courtney Paquette. Subgradient methods for sharp weakly convex functions. J. Optim. Theory Appl. (179) (2018), no. 3, 962-982, https://doi.org/10.1007/s10957-018-1372-8
- Damek Davis, Dmitriy Drusvyatskiy, Courtney Paquette. The nonsmooth landscape of phase retrieval. IMA J. Numer. Anal. 40 (2020), no. 4, 2652-2695, https://doi.org/10.1093/imanum/drz031
- Courtney Paquette, Hongzhou Lin, Dmitriy Drusvyatskiy, Julien Mairal, Zaid Harchaoui. Catalyst Acceleration for Gradient-Based Non-Convex Optimization. 22nd International Conference on Artificial Intelligence and Statistics (AISTATS) 2018, http://proceedings.mlr.press/v84/paquette18a.html
- Dmitriy Drusvyatskiy, Courtney Paquette. Efficiency of minimizing compositions of convex functions and smooth maps. Math. Program. 178 (2019), no. 1-2, Ser. A, 503-558, https://doi.org/10.1007/s10107-018-1311-3
- Dmitriy Drusvyatskiy, Courtney Paquette. Variational analysis of spectral functions simplified. J. Convex Analysis. 25 (2018), no. 1, 119-134, https://arxiv.org/pdf/1506.05170
EXPOSITORY WRITING
Survey papers based on research projects.
- Courtney Paquette, Elliot Paquette. High-dimensional Optimization. SIAM Views and News 30 (2022), 20:16pp, https://siagoptimization.github.io/assets/views/ViewsAndNews-30-1.pdf
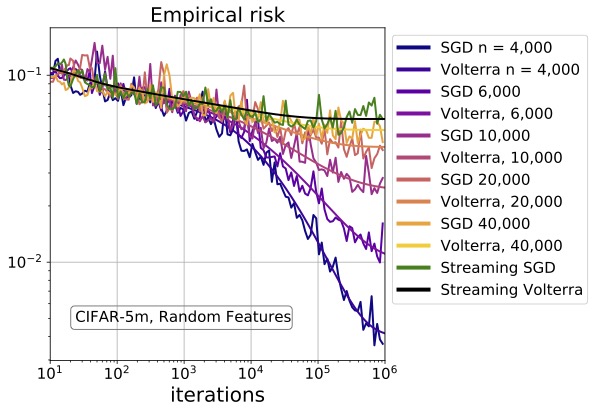
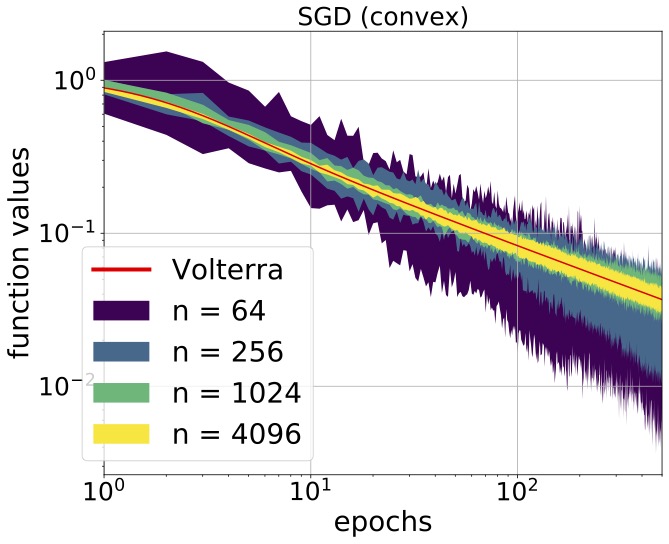
High-dimensional Analysis of Optimization Algorithms
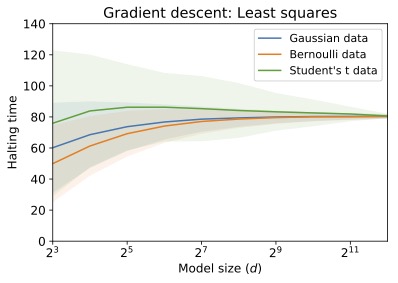
Random Matrix Theory & Machine Learning
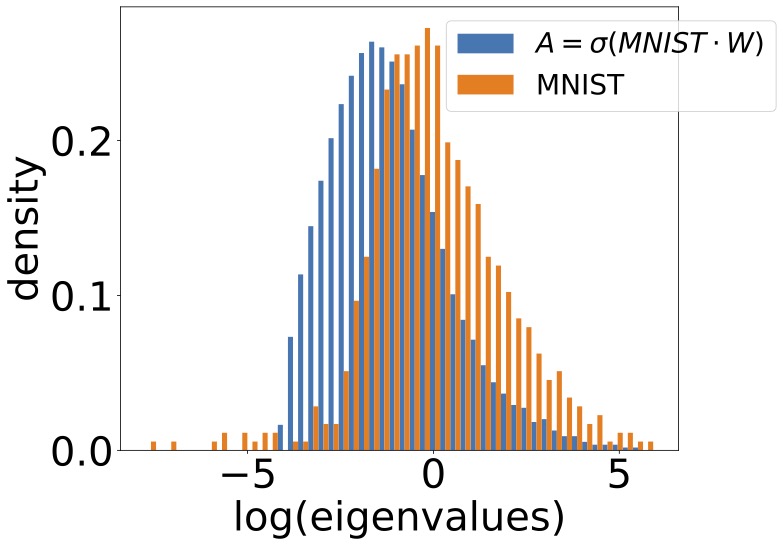
Stochastic Optimization
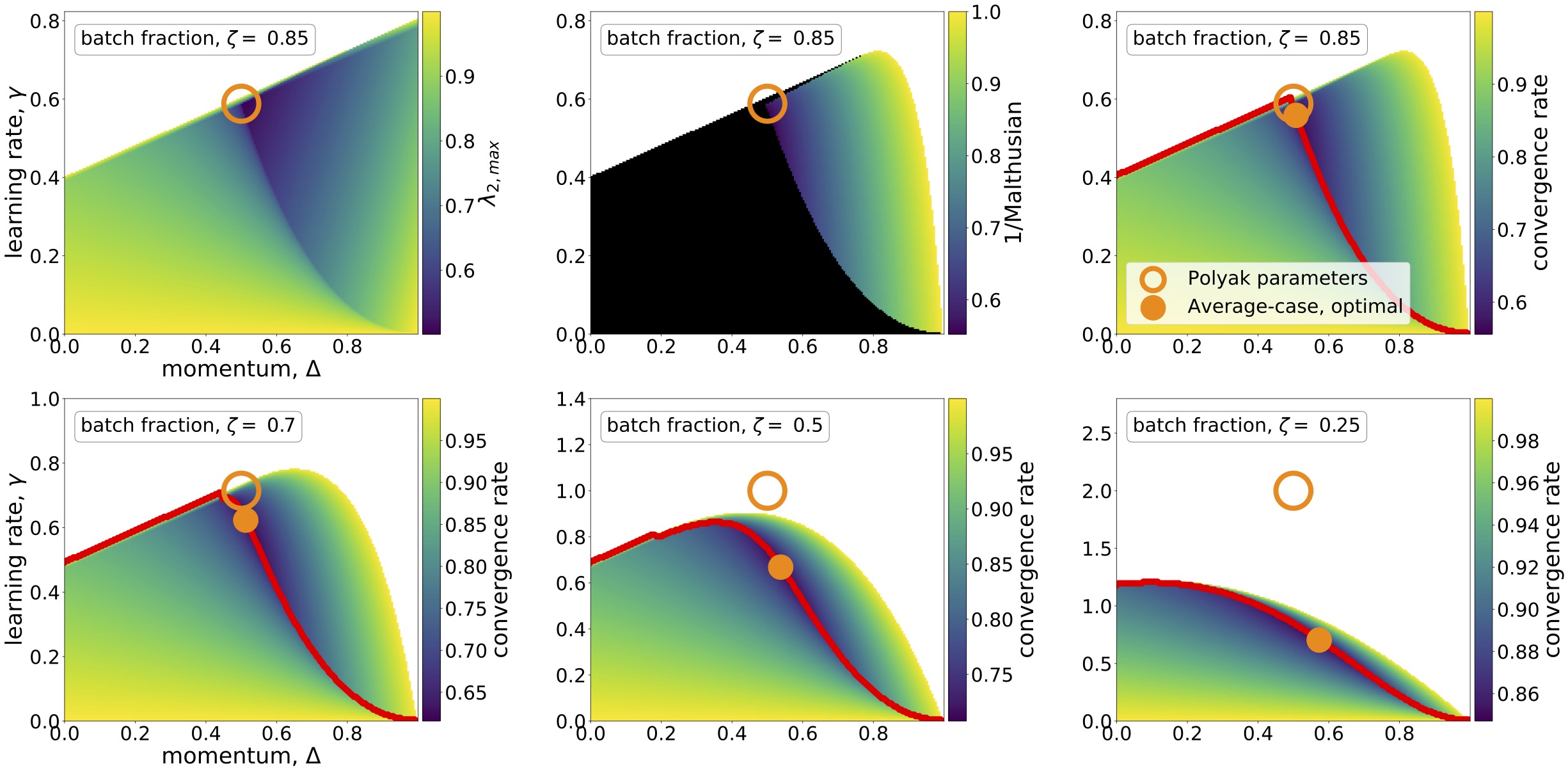
Nonsmooth & Nonconvex
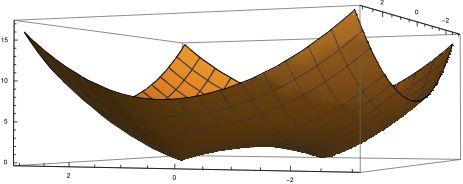
STUDENTS
MSc Students
- Matt Chaubet, 2024-present
- Yixi Wang, 2024-present
- Andrew Mackenzie, 2023-present; (expected grad. 2025)
- Hugo Latourelle-Vigeant, 2022-2024; (PhD, Yale starting Fall 2024); Website: https://hugol-v.github.io/
- Andrew N. Cheng, 2021-2023; (PhD, Harvard); Google Scholar
PhD Students
- Begoña García Malaxechebarría, 2023-present; Website: https://begogar99.github.io/
- Kiwon Lee, 2020-2023; (Lecturer at McGill U.)
Post Docs
- Inbar Seroussi, 2024; (assistant prof. at Tel Aviv U.); Website: https://sites.google.com/view/inbar-seroussi/home
- Elizabeth Collins-Woodfin, 2022-present; (assistant prof. at Oregon starting 2025); Website: https://sites.google.com/view/e-collins-woodfin
- Yakov Vaisbourd, 2020-2022;
Google Interns
- Tomás González, 2022-2023; (PhD, Carnegie Mellon); Google Scholar
- Konstantin Mishchenko, 2021; (research scientist at Samsung AI); Website: https://www.konstmish.com/
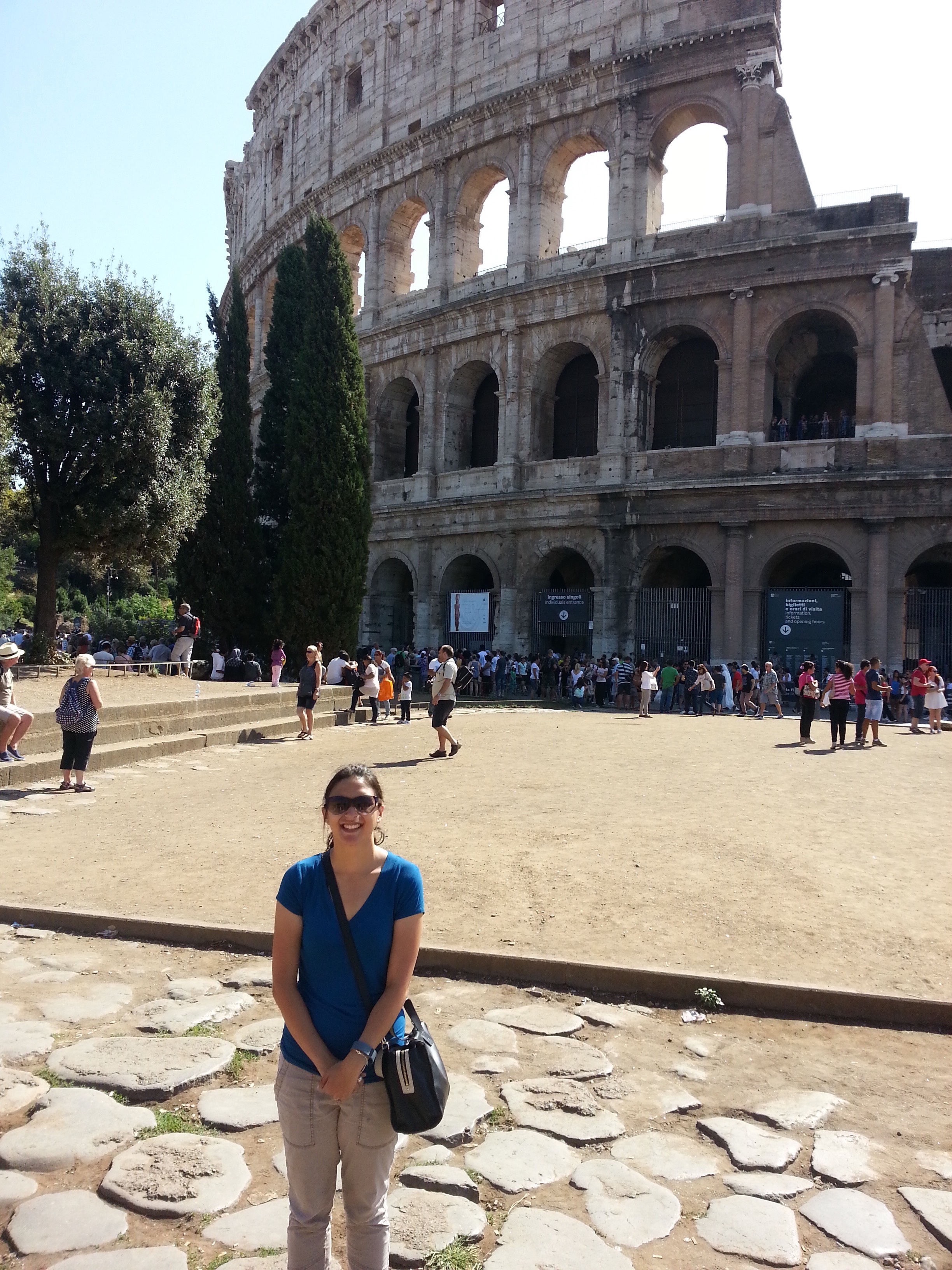
PRESENTATIONS
I have given talks on the research above at the following conferences.
COLLOQUIUM/PLENARY SPEAKER
- Math Department Colloquium , University of Washington, Seattle, WA, November 2023
- Math Department Colloquium , Rensselaer Polytechnic Institute, Troy, NY, January 2023
- Plenary speaker, Conference on the Mathematical Theory of Deep Neural Networks , Deep Math, UC San Diego, CA, November 2022
- Information Systems Laboratory Colloquium, Stanford University, October 2022
- Plenary speaker, GroundedML Workshop 10th International Conference on Learning Representations (ICLR), (virtual event), April 2022
- Courant Institute of Mathematical Sciences Colloquium, New York University (NYU), New York, NY (virtual event), January 2022
- Mathematics Department Colloquium, University of California-Davis, Davis, CA (virtual event), January 2022
- Operations Research and Financial Engineering Colloquium, Princeton University, Princeton, NJ (virtual event), January 2022
- Computational and Applied Mathematics (CAAM) Colloquium, Rice University, Houston, TX, December 2021
- Plenary speaker, Beyond first-order methods in machine learning systems Workshop, International Conference on Machine Learning (ICML), (virtual event) July 2021
- Operations Research Center Seminar, Sloan School of Management, Massachusetts Institute of Technology (MIT), Boston, MA (virtual event) February 2021
- Operations Research and Information Engineering (ORIE) Colloquium, Cornell University, Ithaca, NY (virtual event) February 2021
- Tutte Colloquium, Combinatorics and Optimization Department, University of Waterloo, Waterloo, ON (virtual event) June 2020
- Center for Artificial Intelligence Design (CAIDA) colloquium, University of British Columbia, Vancouver, BC (virtual event) June 2020
- Math Department Colloquium, Ohio State University, Columbus, OH, February 2019
- Applied Math Colloquium, Brown University, Providence, RI, February 2019
- Mathematics and Statistics Colloquium, St. Louis University, St. Louis, MO, November 2019
INVITED TALKS
- Invited speaker, Portuguese American Optimization Workshop (PAOW), Azores, June 2025 (upcoming)
- Invited speaker, Physics of AI Algorithms, Les Houches, France, January 2025 (upcoming)
- Invited speaker, Computational Harmonic Analysis in Data Science and Machine Learning Workshop, Oaxaca, Mexico, September 2024 (upcoming)
- Parameter-Free Optimization (invited speaker), 25th International Symposium on Mathematical Programming (ISMP), Montreal, QC, July 2024 (upcoming)
- Continuous optimization lecture, CIFAR Deep Learning + Reinforcement Learning Summer School, Toronto, ON, July 2024 (upcoming)
- DIMACS Workshop on Modeling Randomness in Neural Network Training, Rutgers University, New Brunswick, NJ, June 2024 (upcoming)
- Invited speaker, CIFAR AI CAN, Banff, AB, May 2024
- Youth in High-dimensions, The Abdus Salam International Centre for Theoretical Physics, Trieste, Italy, May 2024
- Harvard Probability Seminar Series, Harvard University, Cambridge, MA, April 2024
- Math Machine Learning Seminar, UCLA, (virtual) March 2024
- INTER-MATH-AI Monthly Seminar, U. of Ottawa, Ottawa, ON, February 2024
- The Mathematics of Data Science, Institute for Mathematical Sciences (IMS), Singapore, January 2024
- Optimization and Algorithm Design Workshop, Simons Institute for the Theory of Computing, Berkeley, CA, December 2023
- Midwest Optimization Meeting, University of Michigan, Ann Arbor, MI, November 2023
- MTL-OPT Seminar, MILA, Montreal, QC, October 2023
- Invited speaker, CodEx Seminar, (virtual) October 2023
- Optimization Seminar, University of Pennsylvania, Philadelphia, PA, September 2023
- Invited speaker, Foundations of Computational Mathematics, Paris, France, June 2023
- Invited speaker, SIAM Conference on Optimization, Seattle, WA, June 2023
- DACO Seminar, ETH Zurich, Zurich, Switzerland, April 2023
- Invited speaker, US-Mexico Workshop on Optimization and Its Applications (In honor of Steve Wright’s 60th Birthday Conference), Oaxaca, Mexico, January 2023
- Department of Decision Sciences Seminar, HEC, Montreal, QC, December 2022
- Dynamical Systems Seminar, Brown University, Providence, RI, October 2022
- Tea Talk, Quebec Artificial Intelligence Institute (MILA), Montreal, QC, September 2022
- Adrian Lewis’ 60th Birthday Conference (contributed talk), University of Washington, Seattle, WA, August 2022
- Stochastic Optimization Session (contributed talk), International Conference on Continuous Optimization (ICCOPT 2022), Lehigh University, Bethlehem, PA, July 2022
- Conference on random matrix theory and numerical linear algebra (contributed talk), University of Washington, Seattle, WA, June 2022
- Dynamics of Learning and Optimization in Brains and Machines, UNIQUE Student Symposium, MILA, Montreal, QC, June 2022
- The Mathematics of Machine Learning, Women and Mathematics, Institute of Advanced Study, Princeton, NJ, May 2022
- Robustness and Resilience in Stochastic Optimization and Statistical Learning: Mathematical Foundations, Ettore Majorana Foundation and Centre for Scientific Culture, Erice, Italy, May 2022
- Optimization in Data Science (contributed talk), INFORMS Optimization Society Meeting 2022, Greenville, SC, March 2022
- Optimization and ML Workshop (contributed talk), Canadian Mathematical Society (CMS), Montreal, QC, December 2021
- Operations Research/Optimization Seminar, UBC-Okanagan and Simon Fraser University, Burnaby, BC, December 2021
- Machine Learning Advances and Applications Seminar, Fields Institute for Research in Mathematical Sciences, Toronto, ON, November 2021
- Methods for Large-Scale, Nonlinear Stochastic Optimization Session (contributed talk), SIAM Conference on Optimization, Spokane, WA, July 2021
- MILA TechAide AI Conference (invited talk), Montreal, QC, May 2021
- Minisymposium on Random matrices and numerical linear algebra (contributed talk), SIAM Conference on Applied Linear Algebra,, virtual event, May 2021
- Numerical Analysis Seminar (invited talk), Applied Mathematics, University of Washington, Seattle, WA, April 2021
- Applied Mathematics Seminar (invited talk), Applied Mathematics, McGill University, Montreal, QC, January 2021
- Optimization and ML Workshop (contributed talk), Canadian Mathematical Society (CMS), Montreal, QC, December 2020
- UW Machine Learning Seminar (invited talk), Paul G. Allen School of Computer Science, University of Washington, Seattle, WA, November 2020
- Soup and Science (contributed talk), McGill University, Montreal, QC, September 2020
- Conference on Optimization, Fields Institute for Research in Mathematical Science, Toronto, ON, November 2019
- Applied Math Seminar, McGill University, Montreal, QC, February 2019
- Applied Math and Analysis Seminar, Duke University, Durham, NC, January 2019
- Google Brain Tea Talk, Google, Montreal, QC, January 2019
- Young Researcher Workshop, Operations Research and Information Engineering (ORIE), Cornell University, Ithaca, NY, October 2018
- DIMACS/NSF-TRIPODS conference, Lehigh University, Bethlehem, PA, July 2018
- Session talk, INFORMS annual meeting, Houston, TX, October 2017
- Optimization Seminar, Lehigh University, Bethlehem, PA, September 2017
- Session talk, SIAM-optimization, Vancouver, BC, May 2017
- Optimization and Statistical Learning, Les Houches, April 2017
- West Coast Optimization Meeting, University of British Columbia (UBC), Vancouver, BC, September 2016
SUMMER SCHOOLS & TUTORIALS
- High-dimensional Learning Dynamics with Applications to Random Matrices, Random Matrices and Scaling Limits Program, Mittag Leffler, Stockholm, Sweden, October 2024 (upcoming)
- Nonconvex and Nonsmooth Optimization Tutorial, East Coast Optimization Meeting, George Mason University, Fairfax, VA, April 2022
- Average Case Complexity Tutorial, Workshop on Optimization under Uncertainty, Centre de recherches mathematiques (CRM), Montreal, QC, September 2021
- Stochastic Optimization, Summer School talk for University of Washington’s ADSI Summer School on Foundations of Data Science, Seattle, WA, August 2019
WORKSHOPS & TUTORIALS
Workshops
I have had the pleasure to organize some wonderful optimization workshops. Please consider submitting papers to these great organizations.- Optimization for Machine Learning Workshop, NeurIPS • Program Chair (2020,2021,2022,2023) • Annual event in early December, late November • Website: https://opt-ml.org/ • Accepts papers starting in July (see website for details)
- High-dimensional Learning Dynamics (HiLD) Workshop, ICML • Program Chair (2023,2024) • Held at ICML in mid-July • Website: see here • Accepts papers starting in March (see website for details)
- Montreal AI Symposium
• Program Chair (2021) • Annual event in early September-October • Website: http://montrealaisymposium.com/ • Accepts papers starting in June (see website for details); Must be connected to the greater Montreal area
Tutorials
I have organized the following tutorials based on my research. For more information, please see the corresponding website.
- Random Matrix Theory and Machine Learning Tutorial as part of ICML • 2021 • Website:https://random-matrix-learning.github.io/ • 3 hour introductory tutorial on applying random matrix theory techniques in machine learning
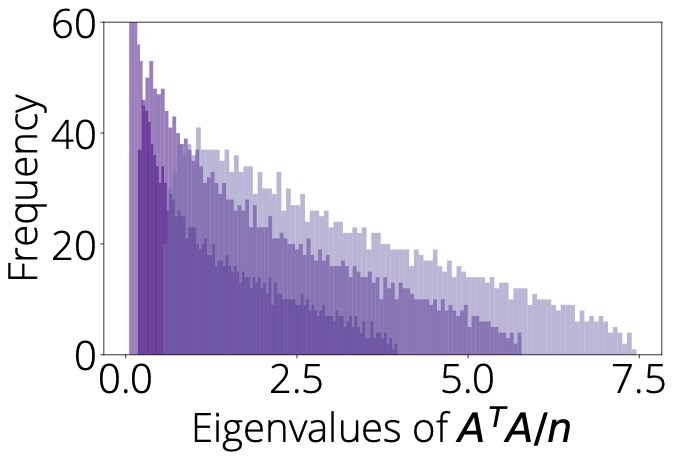
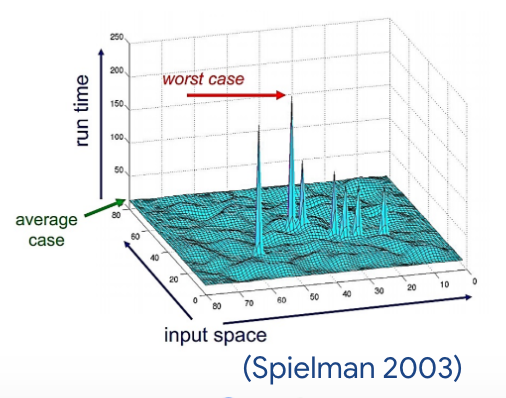
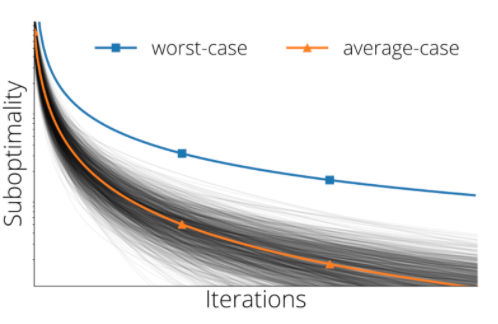
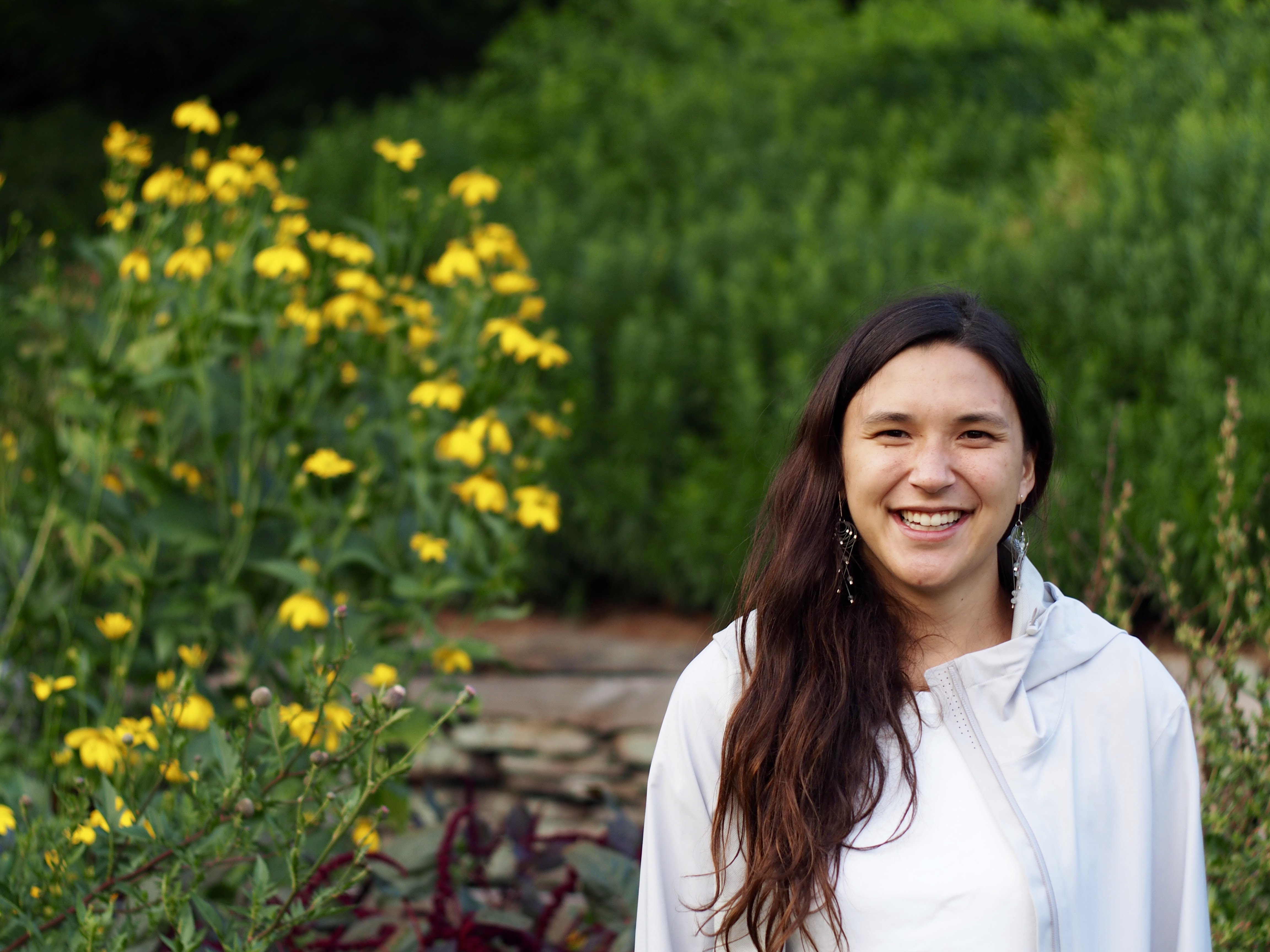
TEACHING
Past Courses
I have taught the following courses:
- McGill University, Mathematics and Statistics Department
- Math 560 (graduate, instructor): Numerical Optimization, Winter 2021, Winter 2022
- Math 315 (undergraduate, instructor): Ordinary Differential Equations, Fall 2020, Fall 2021
- Math 597 (graduate, instructor): Topics course on Convex Analysis and Optimization, Fall 2021
- Math 417/517 (advanced undergraduate/graduate, instructor): Linear Programming, Fall 2022
- Math 463/563 (advanced undergraduate/graduate, instructor): Convex Optimization, Winter 2023, Winter 2024
- Lehigh University, Industrial and Systems Engineering
- ISE 417 (graduate, instructor): Nonlinear Optimization, Spring 2018
- University of Washington, Mathematics Department
- Math 125 BC/BD (undergraduate, TA): Calculus II Quiz Section, Winter 2017
- Math 307 E (undergraduate, instructor): Intro to Differential Equations, Winter 2016
- Math 124 CC (undergraduate, TA): Calculus 1, Autumn 2015
- Math 307 I (undergraduate, instructor): Intro to Differential Equations, Spring 2015
- Math 125 BA/BC (undergraduate, TA): Calculus 2, Winter 2015
- Math 307 K (undergraduate, instructor): Intro to Differential Equations, Autumn 2014
- Math 307 L (undergraduate, instructor): Intro to Differential Equations, Spring 2014
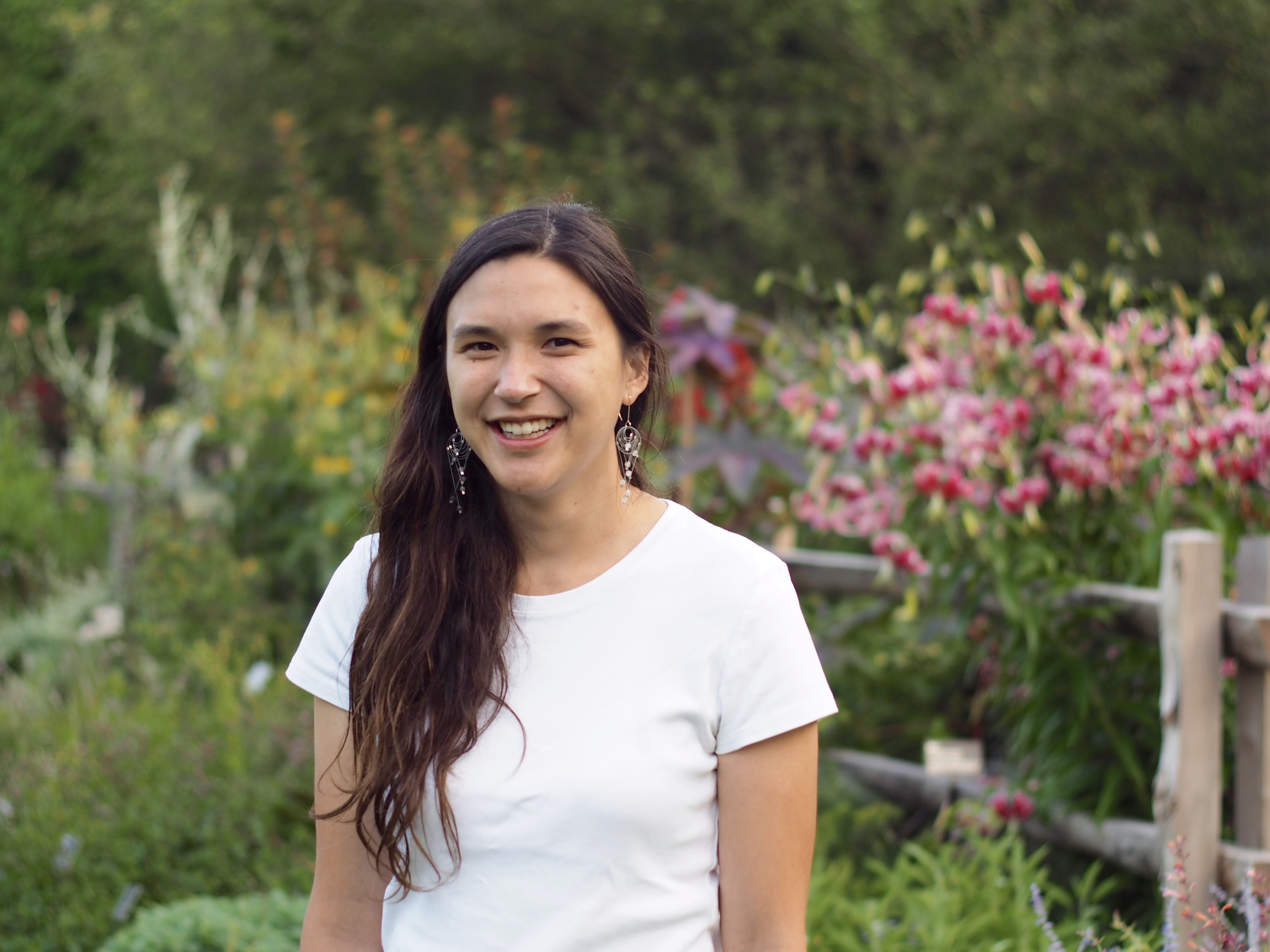
Biosketch (for talks)
Courtney Paquette is an assistant professor at McGill University and a CIFAR Canada AI chair, MILA. She was awarded a Sloan Fellowship in Computer Science in 2024. Paquette’s research broadly focuses on designing and analyzing algorithms for large-scale optimization problems, motivated by applications in data science. She is also interested in scaling limits of stochastic algorithms. She received her PhD from the mathematics department at the University of Washington (2017), held postdoctoral positions at Lehigh University (2017-2018) and University of Waterloo (NSF postdoctoral fellowship, 2018-2019), and works 20% as a research scientist at Google DeepMind, Montreal.
Research currently supported by CIFAR AI Chair, MILA; NSERC Discovery Grant; FRQNT New university researcher’s start-up program; Sloan Fellowship.
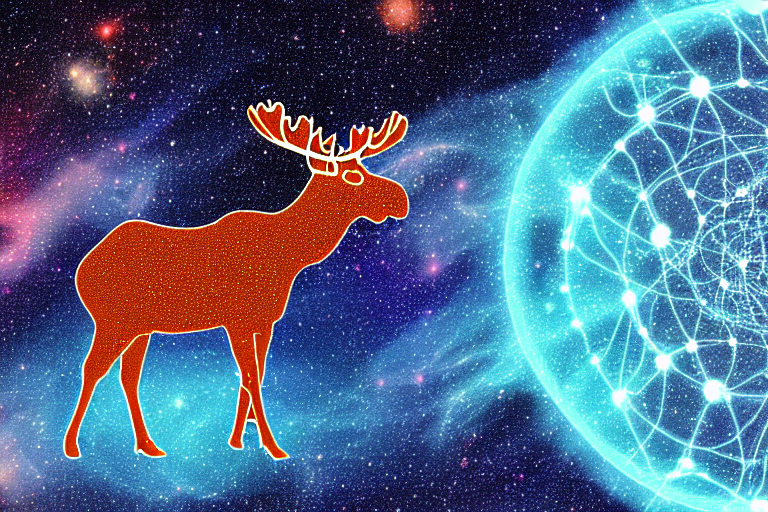
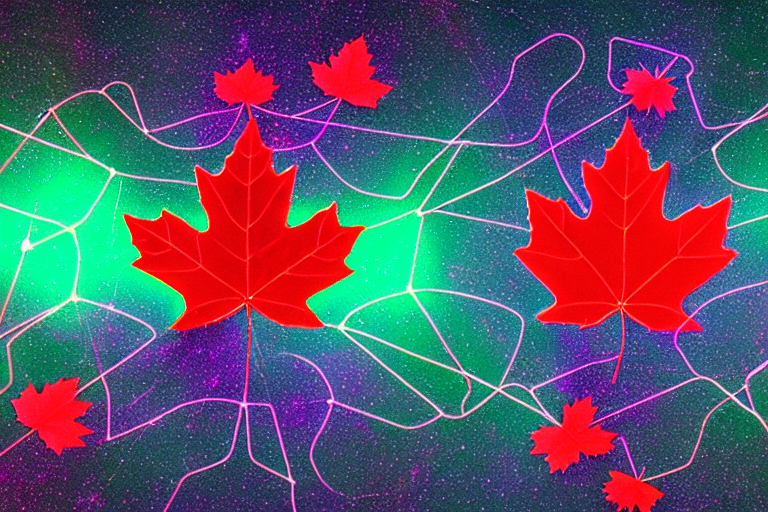
McGill University:
Random Matrix Theory & Machine
Learning & Optimization Graduate Seminar (RMT+ML+OPT
Seminar)
Current Information, Fall 2024 TBD
All are welcome to attend (in person) at McGill University.
For a complete schedule, see Website
- Website: see here
- WHEN: TBD
- WHERE: TBD